Ambient Sensing & Naturalistic Driving Systems for Proactive School Safety Management
With the advent of seemingly unstructured big data, and through seamless integration of computation and physical components, cyber-physical systems (CPS) provide an innovative way to enhance safety and resiliency of transport infrastructure. This study focuses on real-world microscopic driving behavior and its relevance to school zone safety – expanding the capability, usability, and safety of dynamic physical systems through data analytics. Driving behavior and school zone safety is a public health concern. The sequence of instantaneous driving decisions and its variations prior to involvement in safety critical events, defined as driving volatility, can be a leading indicator of safety. By harnessing unique naturalistic data on more than 41,000 normal, crash, and near-crash events featuring over 9.4 million temporal samples of real-world driving, a characterization of volatility in microscopic driving decisions is sought at school and non-school zone locations.
A big data analytic methodology is proposed for quantifying driving volatility in microscopic real-world driving decisions. Eight different volatility measures are then linked with detailed event-specific characteristics, health history, driving history/experience, and other factors to examine crash propensity at school zones. A comprehensive yet fully flexible state-of-the-art generalized mixed logit framework is employed to fully account for distinct yet related methodological issues of scale and random heterogeneity, containing multinomial logit, random parameter logit, scaled logit, hierarchical scaled logit, and hierarchical generalized mixed logit as special cases. The results reveal that both for school and non-school locations, drivers exhibited greater intentional volatility prior to safety-critical events.
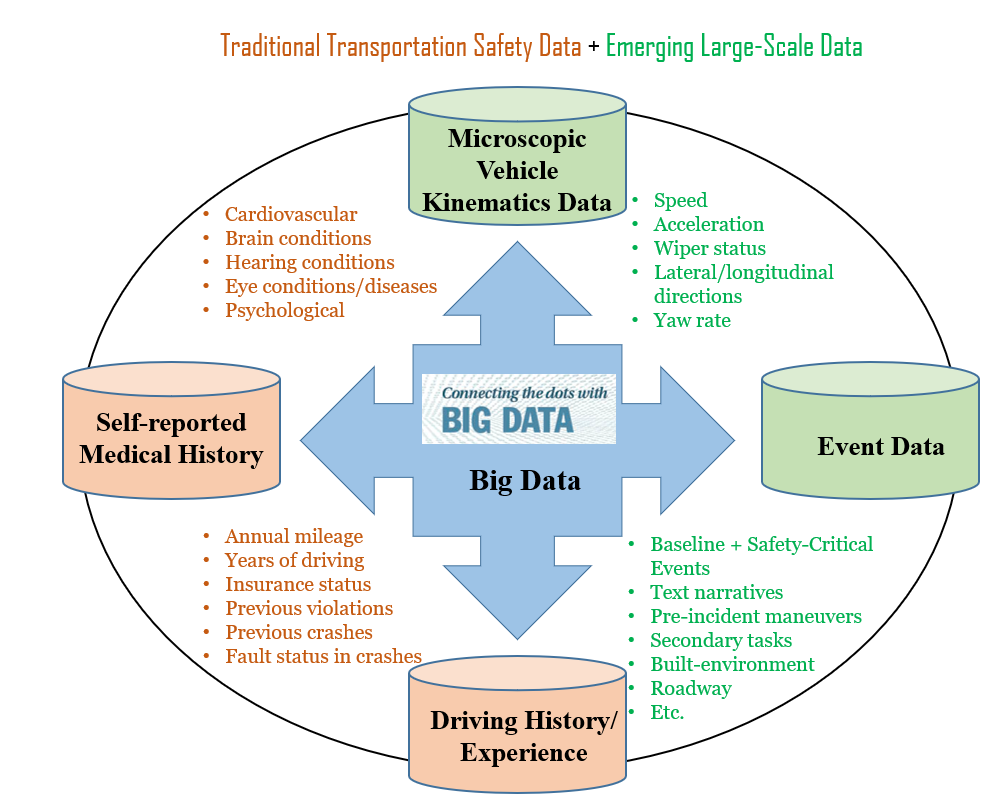
Evidence is found that an increase in volatility in positive and negative vehicular jerk in longitudinal and lateral direction increases the probability of unsafe outcomes (crashes or near-crashes) at school zones. A one-unit increase in intentional volatility associated with positive vehicular jerk in longitudinal direction increases the probability of crash outcome by 0.0528 units. Importantly, the effect of negative vehicular jerk (braking) in longitudinal direction on the likelihood of crash outcome is almost double.
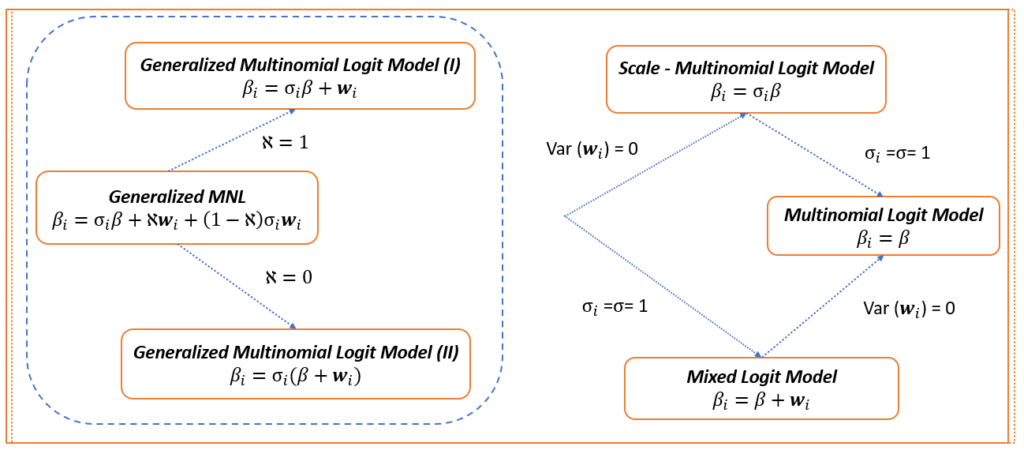
Methodologically, Hierarchical Generalized Mixed Logit model resulted in best-fit, simultaneously accounting for scale and random heterogeneity. When accounted for separately, more parsimonious models accounting for scale heterogeneity performed comparably to the less parsimonious counterparts accounting for random heterogeneity. Importantly, even after accounting for random heterogeneity, substantial heterogeneity due to a “pure scale-effect” is still observed, underscoring the importance of scale effects in influencing the overall contours of variations in modeled relationships. The study demonstrates the value of observational study design and big data analytics for understanding extreme driving behaviors in safe vs. unsafe driving outcomes at vulnerable locations. Implications for designing personalized school zone behavioral countermeasures are discussed.
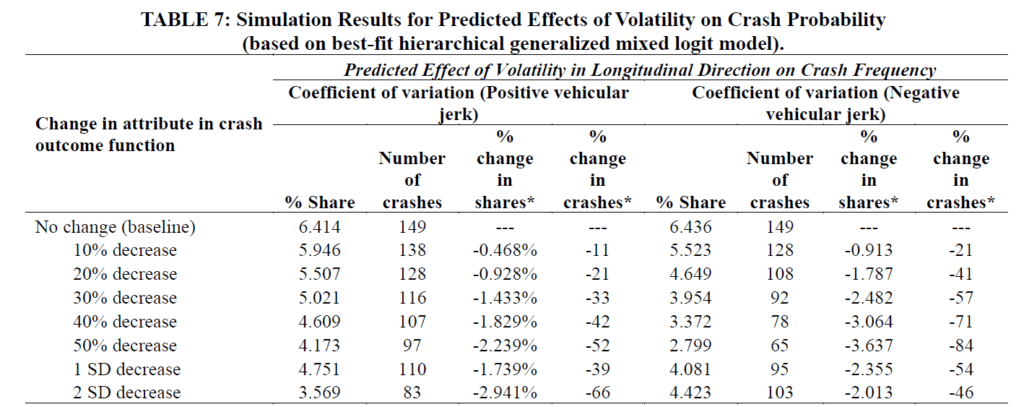
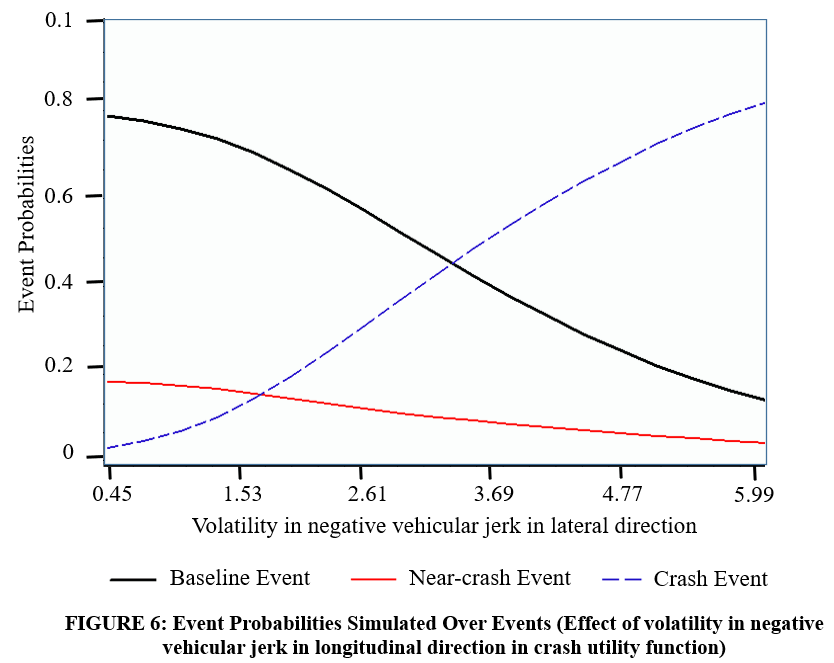
Publications:
Wali, B., & Khattak, A. J. (2020). Harnessing ambient sensing & naturalistic driving systems to understand links between driving volatility and crash propensity in school zones–A generalized hierarchical mixed logit framework. Transportation research part C: emerging technologies, 114, 405-424.
Wali, B., A.J. Khattak (2018). Is Driving Volatility in School Zones Related to Crash Propensity in a Naturalistic Driving Environment? A Deep Learning and Generalized Mixed Discrete Choice Framework with Observed and Unobserved Heterogeneity (Invited Presentation: Second Strategic Highway Research Program Safety Data Bonanza), Presented at the 98th Annual Meeting of the Transportation Research Board, Washington DC, USA.
Major Contributors:
Asad J. Khattak (PI & advisor), Ph.D., Beaman Distinguished Professor, University of Tennessee, Knoxville.
Acknowledgements:
Sponsorship and funding for this project was provided by the Student Paper Competition: SHRP 2 Safety Data Bonanza through an award granted to B. Wali as the primary author and A. Khattak as contributory author/supervisor. The sponsorship of U.S. National Academy of Sciences in funding the first author’s participation in the 2019 TRB Annual Meeting for presenting the findings of an earlier version of this paper is greatly appreciated. The assistance of Virginia Tech Transportation Institute (VTTI) data stewards’ team in answering all relevant queries is acknowledged. The valuable contribution of Mr. Numan Ahmad in assisting with writing the statistical methodology (section 2.3) is also sincerely acknowledged. The authors appreciate the support provided by Ms. Whitney Atkins (VTTI) in executing the Data Use License Agreement and generously providing the breakdown of sampled events across U.S. states. Finally, the authors are thankful to two anonymous reviewers for providing several useful comments. Any opinions, findings, and conclusions or recommendations expressed in this paper are solely those of the authors.