Study of Driving Volatility in Connected & Cooperative Vehicle Systems
This project focused on combining connected vehicles data, naturalistic driving sensor and telematics data, and traditional transportation data to prospect opportunities for engineering smart and proactive transportation systems. The key idea behind the dissertation is to understand (and where possible reduce) “driving volatility” in instantaneous driving decisions and increase driving and locational stability. As a new measure of micro driving behaviors, the concept of “driving volatility” captures the extent of variations in driving, especially hard accelerations/braking, jerky maneuvers, and frequent switching between different driving regimes. The key motivation behind analyzing driving volatility is to help predict what drivers will do in the short term.
Consequently, we developed a “volatility matrix” which takes a systems approach to operationalizing driving volatility at different levels, trip-based volatility, location-based volatility, event-based volatility, and driver-based volatility. At the trip-level, the dynamics of driving regimes extracted from Basic Safety Messages transmitted between connected vehicles are analyzed at a microscopic level, and where the interactions between microscopic driving decisions and ecosystem of mapped local traffic states in close proximity surrounding the host vehicle are characterized. Another new idea relates to extending driving volatility to specific network locations, termed as “location-based volatility”. A new methodology is proposed for combining emerging connected vehicles data with traditional transportation data (crash, traffic, road geometrics data, etc.) to identify roadway locations where traffic crashes are waiting to happen. The idea of event-based and driver-based volatility introduces the notion that volatility in longitudinal and lateral directions prior to involvement in safety critical events (crashes/near-crashes) can be a leading indicator of proactive safety.
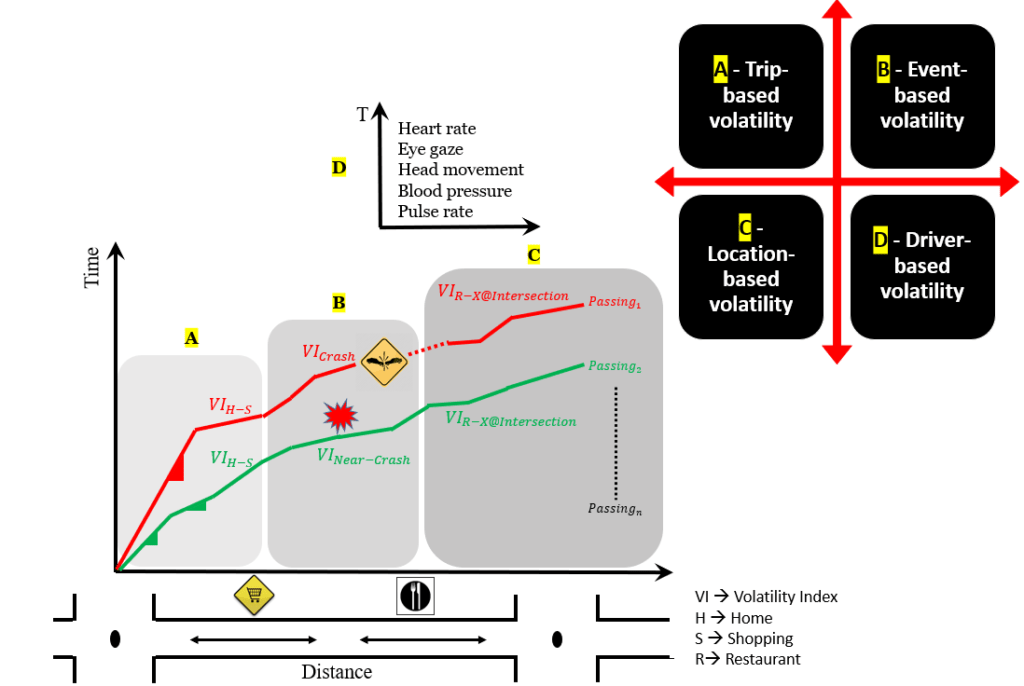
Overall, by studying driving volatility from different lenses, the dissertation contributes to the scientific analysis of real-world connected vehicles data – generating actionable knowledge relevant to the design of smart and intelligent transportation systems. The concept of driving volatility matrix provides a systems framework for characterizing the health of three fundamental elements of a transportation system: health of driver, environment, and the vehicle. The implications of the findings and potential applications to proactive network level screening, customized driver assist and control systems, driving performance monitoring are discussed in detail.
Publications:
Khattak, A. J., & Wali, B. (2017). Analysis of volatility in driving regimes extracted from basic safety messages transmitted between connected vehicles. Transportation research part C: emerging technologies, 84, 48-73.
Wali, B., Khattak, A. J., Bozdogan, H., & Kamrani, M. (2018). How is driving volatility related to intersection safety? A Bayesian heterogeneity-based analysis of instrumented vehicles data. Transportation research part C: emerging technologies, 92, 504-524.
Kamrani, M., Wali, B., & Khattak, A. J. (2017). Can data generated by connected vehicles enhance safety?: Proactive approach to intersection safety management. Transportation Research Record, 2659(1), 80-90.
Wali, B., Khattak, A. J., & Karnowski, T. (2020). The relationship between driving volatility in time to collision and crash-injury severity in a naturalistic driving environment. Analytic methods in accident research, 28, 100136.
Wali, B., Khattak, A. J., & Karnowski, T. (2019). Exploring microscopic driving volatility in naturalistic driving environment prior to involvement in safety critical events—Concept of event-based driving volatility. Accident Analysis & Prevention, 132, 105277.
Major Contributors:
Asad J. Khattak (PI & advisor), Ph.D., Beaman Distinguished Professor, University of Tennessee, Knoxville.
Hamparsum Bozdogan, Ph.D. (Committee Member). TOBY McKenzie Professor of Statistics and Business Analytics, University of Tennessee, Knoxville.
Acknowledgements:
The research is supported by National Science Foundation (Award number: 1538139).